Zero Shot Cross-Lingual Transfer with Multilingual BERT
Fine-tuning BERT for Sentence Embeddings on English NLI Datasets
Sep 24, 2019 · 1163 words · 6 minute read
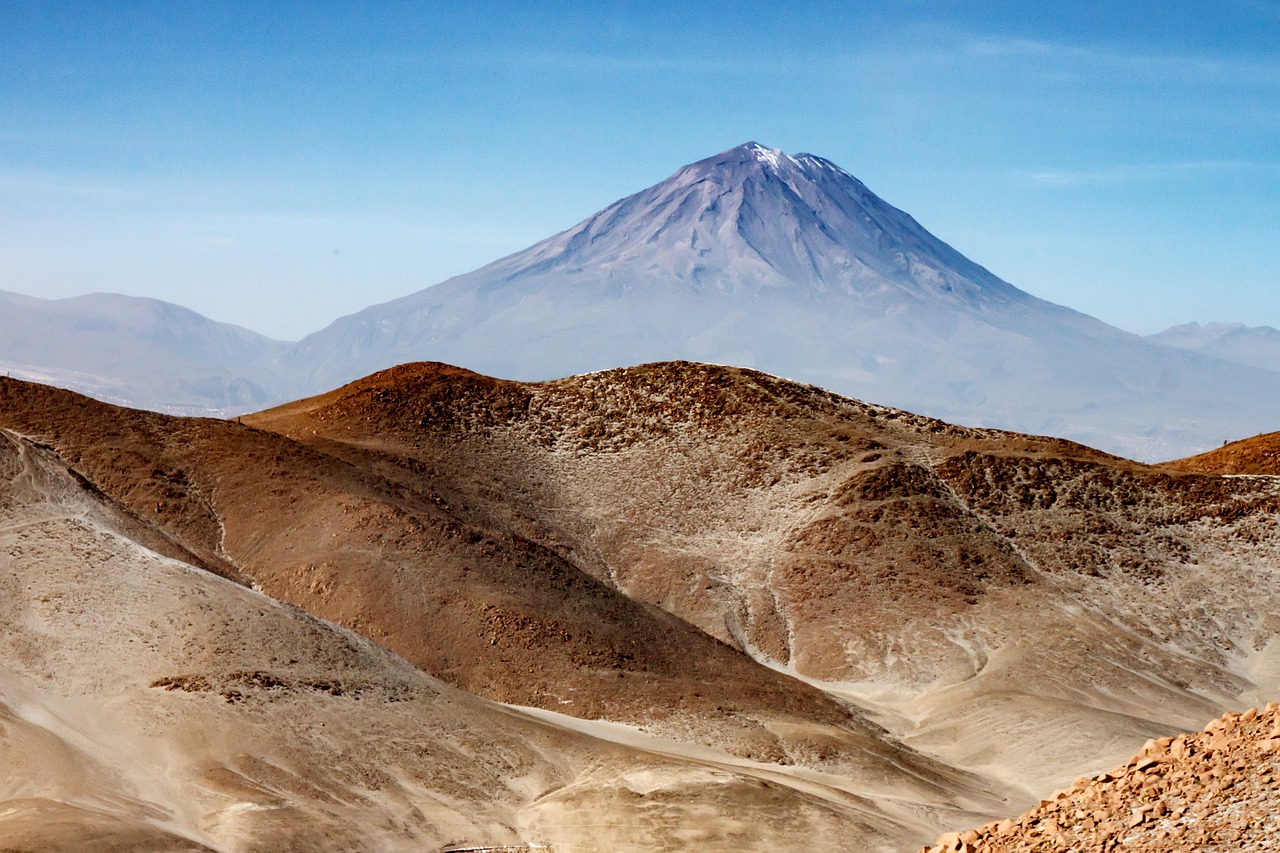
Synopsis
Do you want multilingual sentence embeddings, but only have a training dataset in English? This post presents an experiment that fine-tuned a pre-trained multilingual BERT model(“BERT-Base, Multilingual Uncased” [1][2]) on monolingual(English) AllNLI dataset[4] to create sentence embeddings model(that maps a sentence to a fixed-size vector)[3]. The experiment shows that the fine-tuned multilingual BERT sentence embeddings have generally better performance (i.e. lower error rates) over baselines in a multilingual similarity search task (Tatoeba dataset[5]). However, the error rates are still significantly higher than the ones from specialized sentence embedding models trained with multilingual datasets[5].
Introduction
In this section, we briefly review the technical foundation of the experiment.
BERT
BERT[1] is a language representation model that uses two new pre-training objectives — masked language model(MLM) and next sentence prediction, that obtained SOTA results on many downstream tasks, including some sentence pair classification tasks, such as Natural Language Inference(NLI) and Semantic Textual Similarity(STS).
![BERT is designed to accept one to two sentences/paragraphs as input.[1]](bert_input.png)
BERT is designed to accept one to two sentences/paragraphs as input.[1]
This is how BERT do sentence pair classification — combine two sentences in a row, and take the hidden states of the first token(CLS) to make the classification decision:
![Taken from Figure 3 in [1]](bert_pair_cls.png)
Taken from Figure 3 in [1]
The BERT authors published multilingual pre-trained models in which the tokens from different languages share an embedding space and a single encoder. They also did some experiments on cross-lingual NLI models:
![XNLI results from [2]](bert_multi_nli.png)
XNLI results from [2]
Zero Shot means that the Multilingual BERT system was fine-tuned on English MultiNLI, and then evaluated on the foreign language XNLI test. In this case, machine translation was not involved at all in either the pre-training or fine-tuning.[2]
Their zero-shot configuration is basically what we’re going to use in our experiment.
Sentence-BERT
Although BERT models achieved SOTA on STS tasks, the number of forward-passes needed grows quadratically. It quickly becomes a problem for larger corpora:
Finding in a collection of n = 10,000 sentences the pair with the highest similarity requires with BERT n·(n−1)/2 = 49,995,000 inference computations. On a modern V100 GPU, this requires about 65 hours. [3]
A common solution is to map each sentence to a fixed-size vector living in a vector space where similar sentences are close. Sentence-BERT(SBERT)[3] is a modification of the pre-trained BERT network that does the exact thing. The number of forward-passes needed grows linearly now, making large scale similarity search practical:
This reduces the effort for finding the most similar pair from 65 hours with BERT / RoBERTa to about 5 seconds with SBERT, while maintaining the accuracy from BERT. [3]
In short, SBERT adds an average pooling layer on top of the final layer of BERT to create sentence embeddings, and use the embeddings as input to sub-network targeted at the fine-tuning task.
It also achieves SOTA on multiple tasks when comparing to other sentence embeddings methods.
![The fine-tuning setup (1) and inference setup (2) from [3]](sbert.png)
The fine-tuning setup (1) and inference setup (2) from [3]
For STS and SentEval tasks, SBERT models were fine-tuned on the AllNLI dataset (SNLI + Multi-NLI datasets combined[4]). For supervised STS, SBERT achieves slightly worse results than BERT, and the differences in Spearman correlation are within 3. Overall, SBERT produces very good sentence embeddings.
Experiment
There is a big problem when we try to extend the results of SBERT to other languages — public NLI datasets in other languages are rare, sometimes nonexistent. This experiment tries to find out how the zero-shot cross-lingual transfer learning would work with SBERT and the AllNLI dataset.
Design
We first load the pre-trained bert-base-multilingual-cased
model, and freeze the embedding vectors (otherwise only English vectors will be updated, invalidating vectors in other languages). Then we follow the example training script from the official SBERT Github repo — training_nli.py
to fine-tune the model on the AllNLI dataset for one epoch. All hyper-parameters are the same ones used in the example script.
Results
STS Benchmark
Note the STS Benchmark is in English only. The following statistics are mainly for you to tune the hyper-parameters if you wish to train the model yourself.
Validation set performance:
Cosine-Similarity : Pearson: 0.7573 Spearman: 0.7699
Manhattan-Distance: Pearson: 0.7723 Spearman: 0.7721
Euclidean-Distance: Pearson: 0.7717 Spearman: 0.7719
Dot-Product-Similarity: Pearson: 0.7480 Spearman: 0.7540
Test set performance:
Cosine-Similarity : Pearson: 0.6995 Spearman: 0.7248
Manhattan-Distance: Pearson: 0.7311 Spearman: 0.7258
Euclidean-Distance: Pearson: 0.7307 Spearman: 0.7259
Dot-Product-Similarity: Pearson: 0.6929 Spearman: 0.7024
Tatoeba
The Tatoeba dataset was introduced in [5] as a multilingual similarity search task to evaluate multilingual sentence embeddings. Their open-sourced implementation and pre-trained model — Language-Agnostic SEntence Representations (LASER)[6] achieves very low error rates for high-resource languages[5]:
![Tatoeba error rates reported in [5]](tatoeba_laser.png)
Tatoeba error rates reported in [5]
For our model, the error rates are much higher, mainly because we did not fine-tune on any languages besides English, but we still want the model to have meaningful sentence embeddings for those languages (a.k.a. zero-shot learning).
However, the fine-tuned model reduces the error rates of the baseline models by at most 27%, 50%, and 61% respectively for the mean(taking the average of the hidden states in the final layer), max(taking the maximum in each dimension of the hidden states in the final layer), and CLS(taking the hidden states of the CLS token in the final layer) baselines:
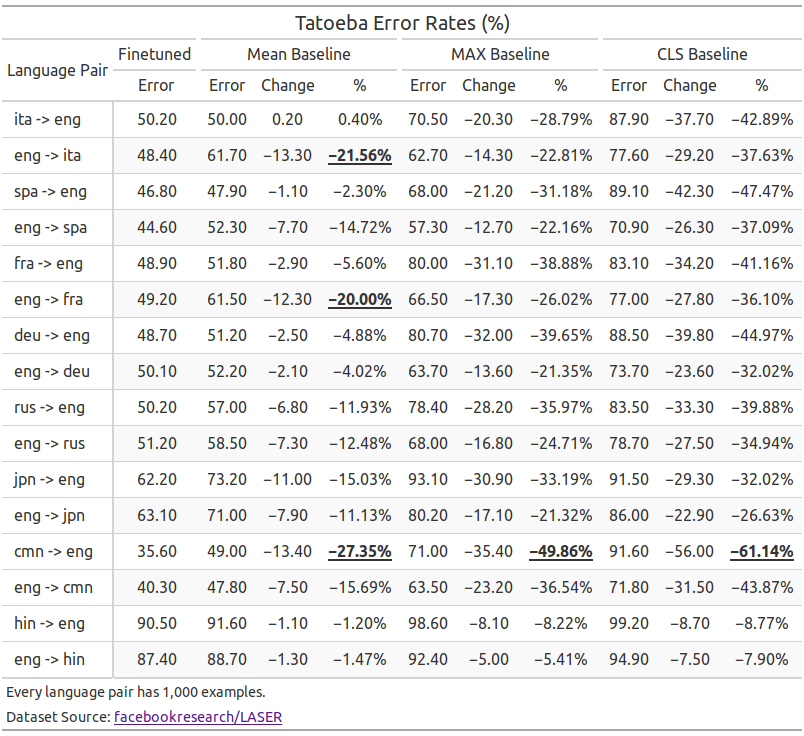
The above results show that English-only fine-tuning successfully pull sentences that are semantically similar but in different languages closer to each other. Although the error rates are still far from ideal, it could be a good starting point and could reduce the amount of data in other languages required to improve the representations of those languages.
Future Work
- Hyper-parameter tuning (e.g. training for more epochs, using different learning rate schedule, etc.).
- Freeze lower layers of the transformer in the multilingual BERT pre-trained model to better preserve the lower level multilingual representations.
- As suggested in bert-as-service[7], using the hidden states from the second-to-last layer could improve the sentence embeddings model. We can try fine-tuning on that layer and compare it with the performance of the baselines that uses hidden states from the same layer.
- Evaluate the Multilingual Universal Sentence Encoders[8][9] on the Tatoeba dataset for comparison.
Source Code
The notebook used for this post is published on Github: Multilingual Bert on NLI.ipynb. I also incorporated the Tatoeba dataset in my fork ceshine/sentence-transformers from UKPLab/sentence-transformers. You should be able to clone the repo and reproduce the results in the notebook. Please report back if you encounter any problems. I might have messed up some of the soft links, but I have not checked them thoroughly.
References
- Devlin, J., Chang, M.-W., Lee, K., & Toutanova, K. (2018). BERT: Pre-training of Deep Bidirectional Transformers for Language Understanding.
- google-research/bert Multilingual README
- Reimers, N., & Gurevych, I. (2019). Sentence-BERT: Sentence Embeddings using Siamese BERT-Networks.
- UKPLab/sentence-transformers NLI Models
- Artetxe, M. (2018). Massively Multilingual Sentence Embeddings for Zero-Shot Cross-Lingual Transfer and Beyond.
- facebookresearch/LASER — Language-Agnostic SEntence Representations.
- hanxiao/bert-as-service: Mapping a variable-length sentence to a fixed-length vector using BERT model.
- Yang, Y., Cer, D., Ahmad, A., Guo, M., Law, J., Constant, N., … Kurzweil, R. (2019). Multilingual Universal Sentence Encoder for Semantic Retrieval.
- Tensorflow Hub - universal-sentence-encoder-multilingual